Author: Xue Wang, Jing Zhang, and C.-C. Jay Kuo
Research Problem
The importance of this research work lies in related morphological characters evaluation for mitochondria objects after accurate object extraction. Studies have shown that the fusion-fission dynamics of mitochondria is involved in many cellular processes, including maintenance of adenosine triphosphate (ATP) levels, redox signaling, oxidative stress generation, and cell death [1-4]. Therefore, mitochondrial morphology can reveal the physiological or pathological status of mitochondria and in a typical analysis, and researchers manually label the mitochondria morphological structures into several subtypes, such as fragmented, networked, and swollen structures [5]. However, although there exist a number of algorithms for mitochondria segmentation [6-8], they require careful manual tuning and optimization while the resultant segmented mitochondria objects are still not correctly classified into standardized morphological subtypes. The challenge is that the gray-level fluorescent intensity is the only clue to segment background from foreground mitochondrial objects.
Main Ideas
To overcome the challenge, our work aims at applying computer vision techniques to achieve accurate segmentation based on texture feature extraction for morphological characters. A 2-stage segmentation system (as shown in Fig. 1) has been built to realize automated mitochondria segmentation.
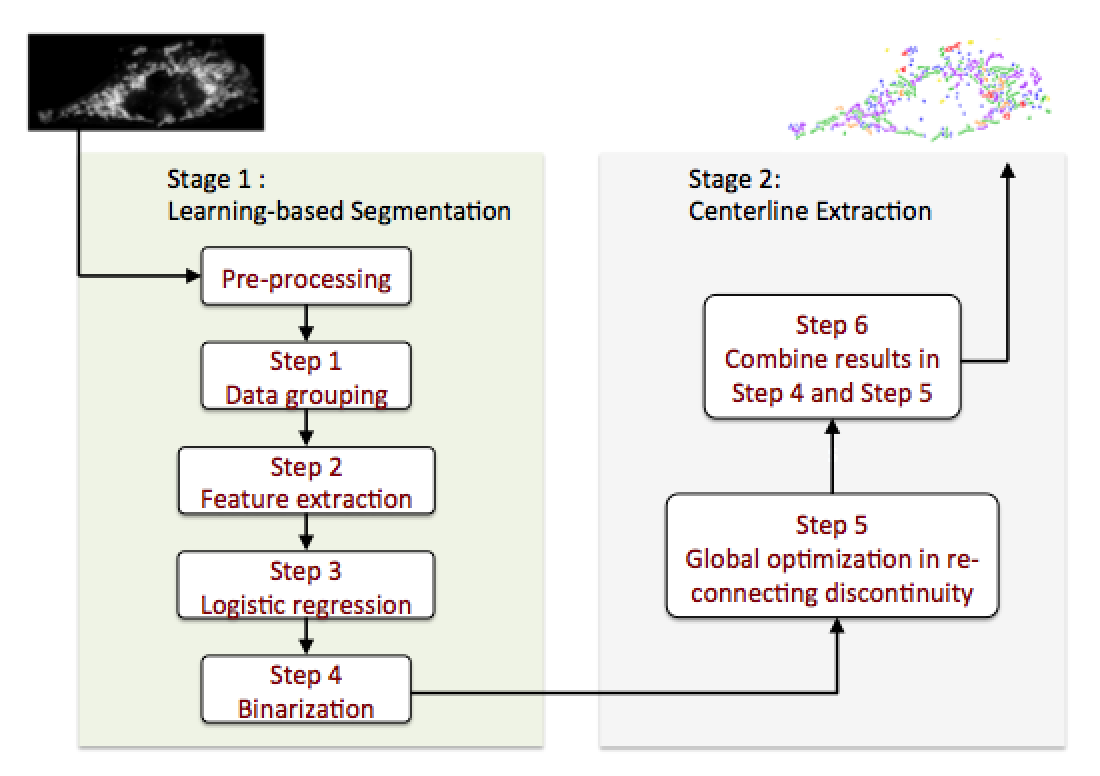
In the Stage I where machine learning classifiers are trained for initial segmentation, the key to the success of this part is that the image signal can be transformed and represented by a linear combination of a subset of extracted texture features, and data grouping methods are applied to enhance the accuracy of classifiers. Our work shows that learning-based approaches fit our problem as they can overcome the existing challenges.
In the Stage II of mitochondria centerline extraction, the cost function is designed based on the human learning/labeling experience to judge the occurrence of connection for each pair of centerline fragments in mitochondria, which improves the accuracy of segmentation and the resultant mitochondrial morphological characteristics.
Innovations
Within all existing segmentation approaches for mitochondria segmentation, Peng’s work [9] explores an approach called Local Adaptive Thresholding (LAT) to define a local neighborhood area of a certain center pixel to do double thresholding, and so is able to reduce the false segmentation due to background noise and intensity variation. The intuition is that the selected local neighborhood area should be large enough to contain both foreground and background pixels but not too large to contain objects far away in the raw images. The proposed algorithm fits for mitochondria fluorescent microscope images than other kinds of imaging schemes, however, it still generates errors in false segmentation when the pixel intensity of foreground mitochondria object is blurred with neighboring background pixels.
Our work involves constant feedback between computation and experimental results, i.e., the approach is built based on combining the principle of computer vision and human vision. The overall segmentation pipeline results in mitochondria segmentation that has morphological features remained as well as segmentation accuracy as high as 98%. Moreover, another advantage is modularity of this pipeline, as each processing step in both two stages in the pipeline could be improved individually to suite different kinds of image dataset for better accuracy.
Demo
Future Challenges
For the future, there are four directions to do.
- Understand the bias among the components of cost function
- Figure out a way to select threshold of cost function more accurately
- Use more data to test the robustness of proposed approach
- Look into the geometric parameters of mitochondria objects
References
- [1] P. Newsholme, et al, “Diabetes associated cell stress and dysfunction: role of mitochondrial and non-mitochondrial ROS production and activity,” 583(1): pp. 9-24, 2007.
- [2] Bonnard C, et al, “Mitochondrial dysfunction results from oxidative stress in the skeletal muscle of diet-induced insulin-resistant mice,” J. Clin. Invest. 118:789-800, 2008.
- [3] Huei-Fen Jheng, et al, “Mitochondrial Fission Contributes to Mitochondrial Dysfunction and Insulin Resistance in Skeletal MuscleMol,” Cell. Biol., 15: 309-319, January 2012.
- [4] Charlotte Brøns, et al, “Effects of high-fat overfeeding on mitochondrial function, glucose and fat metabolism, and adipokine levels in low-birth-weight subjects,” Am. J. Physiol. Endocrinol. Metab., E43-E51, 2011.
- [5] Reis Y, Bernardo-Faura M, Richter D, Wolf T, Brors B, et al, “Multi-Parametric Analysis and Modeling of Relationships between Mitochondrial Morphology and Apoptosis,” PLoS ONE 7(1): e28694, 2012.
- [6] Richard J Giuly, Maryann E Martone and Mark H Ellisman, “Method: automatic segmentation of mitochondria utilizing patch classification, contour pair classification, and automatically seeded level sets,” BMC Bioinformatics, 13:29, 2012.
- [7] Mumcuoglu EU, Hassanpour R, Tasel SF, Perkins G, Martone ME, Gurcan MN, “Computerized detection and segmentation of mitochondria on electron microscope images,” J Microsc., 246(3): 248-65, 2012.
- [8] Aurelien Lucchi, Kevin Smith, Radhakrishna Achanta, Graham Knott, and Pascal Fua, “Supervoxel-Based Segmentation of Mitochondria in EM Image Stacks with Learned Shape Features,” IEEE Transactions on Medical Imaging, 2011.
- [9] Jyh-Ying Peng, Chung-Chih Lin, Chun-Nan Hsu, “Adaptive Image Enhancement for Fluorescence Microscopy,” taai, pp.9-16, 2010 International Conference on Technologies and Applications of Artificial Intelligence, 2010.