Author: Chun-Ting Huang and C.-C. Jay Kuo
Research Problem
“Facial Recognition” has become an important technique to handle the tremendous growing need for identification and verification since last century. The replacement of traditional transaction by electronic transaction successfully gathered attention for facial recognition from research and business communities, because facial recognition requires no physical interaction on behalf of users. The research on facial recognition can be traced back to early 1990s, from the Eigenface proposed by Turk and Pentland in 1991 [1], which has over 11409 citations on Google Scholar. The follow-up development can be concluded into general directions discussed in Face Recognition Vendor Test – FRVT 2002 [2], and different face databases are developed in order to solve various conditions, such as poses, expressions, and environment. A new database called Long Distance Heterogeneous Face Database (LDHF-DB) [3] is focused on face images under various distances and near-infrared camera, which provides an new challenge within this field.
Main Ideas
Since under the long distance, the near-infrared camera can only capture blurred and vague face images, as shown in Fig. 1, causing the template feature’s low performance on LDHF-DB. Therefore, our research only adopts geometric and shape-based features, locally and globally, to determine the input of structured-fusion method. Based on the different characteristics of features we collected from database, we aim to develop an robust classification algorithm with machine learning to distinguish faces under various quality.
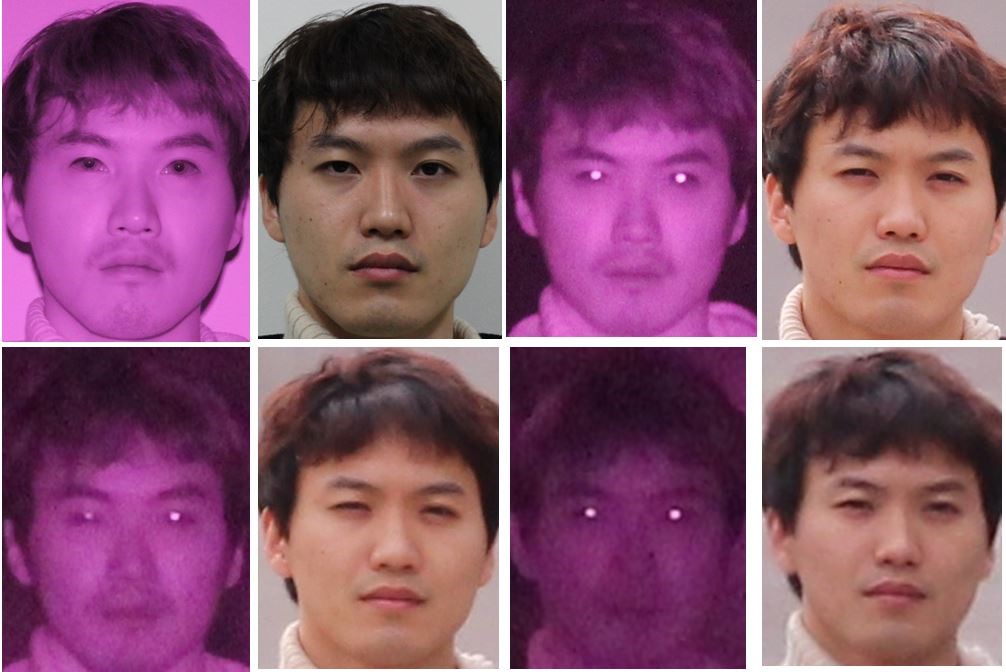
Innovations
The major difference between our work and other research is the feature selection and structured fusion model. I have explained the reason why template method only has a fair performance under the influence of heterogeneous environment. Our proposed model can boost up the recognition rate by adopting different feature’s strength and discarding the outliers for particular set of features, and finally it can utilize the discriminative power between different methods to compromise and deduct the correct result.
Future Challenges
For future work, we will work on other challenge problems that FRVT 2002 has concluded. The first step is to locate and solve a famous challenge, also known as “face in the wild” [4]. Since the current system’s performance is still not reliable under various constraints, there is an ongoing need to improve the current facial recognition system.
References
- [1] M. Turk, A. Pentland, Eigenfaces for Recognition, Journal of Cognitive Neurosicence, Vol. 3, No. 1, Win. 1991, pp. 71-86.
- [2] Phillips, P.J.; Grother, P.; Micheals, R.; Blackburn, D.M.; Tabassi, E.; Bone, M., “Face recognition vendor test 2002,” Analysis and Modeling of Faces and Gestures, 2003. AMFG 2003. IEEE International Workshop on , vol., no., pp.44,, 17 Oct. 2003.
- [3] H. Maeng, S. Liao, D. Kang, S.-W. Lee, and A. K. Jain, “Nighttime Face Recognition at Long Distance: Cross-distance and Cross-spectral Matching”, ACCV, Daejeon, Korea, Nov. 5-9, 2012.
- [4] National Science and Technology Council (NCST) Subcommittee on Biometrics. Face Recognition. 7 August 2006.