Author: Hyunsuk Ko and C.-C. Jay Kuo
Research Problem
A new framework for objective quality evaluation of stereoscopic image pairs is the goal of this research. Quality assessment of stereoscopic image pairs is more complicated than that for 2D images since it is a multi-dimensional problem where the quality is affected by distortion types as well as the relation between the left and right views (e.g. different levels of distortion in two views). In this work, a new formula-based metric was introduced to provide results better than state-of-the art methods. However, the formula-based metric still has its limitation. For further improvement, we further propose a 2-stage fusion model. That is, we classify distortion types into groups and design a set of scorer to handle them separately. In Stage 1, each scorer generates its own score. In Stage 2, all intermediate scores are fused to predict the final quality index with nonlinear regression. Experimental results demonstrate that the proposed quality index outperforms several existing quality assessment methods by a significant margin over different databases.
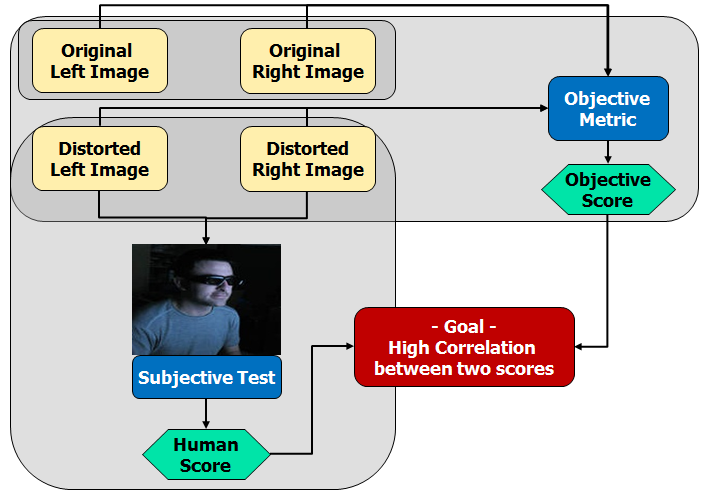
Although research on advanced image/video quality index methods that are more consistent with the human visual experience has made a substantial amount of progress in the last decade, the study on 3D image/video quality is still in its early stage. The 3D quality assessment (QA) is a difficult problem since it is affected by 2D image quality, depth perception and visual discomfort such as eye strain or dizziness. It is particularly challenging when the stereoscopic image pair consists of two views with different quality levels (called asymmetric distortion). To address this problem, we need a deeper understanding of the human visual system (HVS), e.g. the binocular combination in stereovision, to build a robust index that can cope with various scenarios.
Main Ideas
- The novel learning-based 2-stage fusion model is proposed, which considers the impacts of combinations of several distortion types on the perceptual quality of a stereoscopic image pair. We use a learning algorithm to generate the model for the predicted quality score, which finds out optimized relationship among the intermediate scores for individual distortion types. Our model is scalable and can be easily extended to consider new distortion types or include state-of-the-art metrics in the future.
- In order to cover a broad range of distortion types systematically, we classify the distortion types into several groups and provide a relevant feature extraction scheme for each group.
- We prove the superiority and the robustness of the proposed metric by extensive experimental results on symmetrically and asymmetrically distorted databases, as well as a cross-database combining two different databases.
Demo and Simulation Results
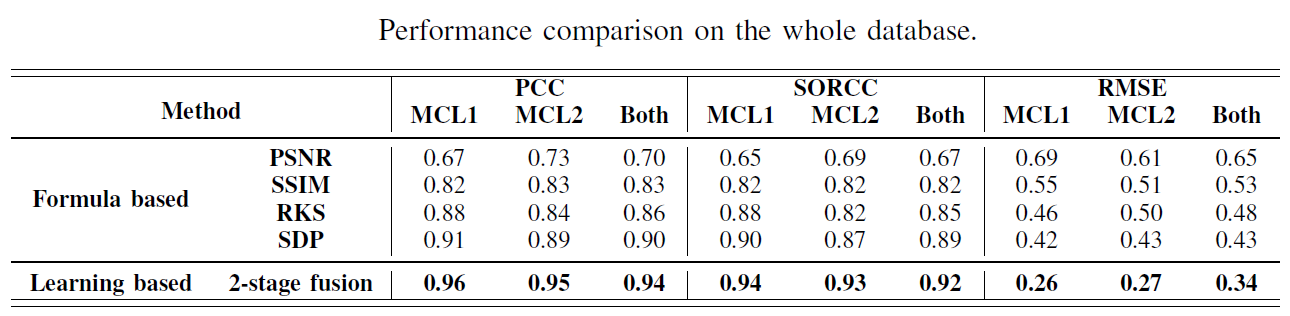
Future Challenges
Our laboratory recently generated the valuable database, so called, MCL3D database with the various rendering distortion types. We confirmed that the performance of all the existing metrics including ours dropped a lot, which means the MCL3D database is the most challenging dataset. Thus, we will improve the current our database to achieve reasonably high performance.
References
- [1] H. Ko, C. Kim, S. Choi, and C.-C. Jay Kuo, “3D image quality index using SDP-based binocular perception model,” accepted to IEEE 11th IVMSP Workshop, Jun. 2013.
- [2] The second work about an Ensemble-based 3D Image quality metric will be submitted to some Journal paper soon.