Author: Kuan-Hsien Liu, Shuicheng Yan, and C.-C. Jay Kuo
Research Problem
Facial image processing has attracted a lot of attention in the computer vision community over the last two decades. The human face can reveal important perceptual characteristics such as the identification, gender, race, emotion, pose, age, etc. Among these characteristics, the age information has its particular importance. The aging progress is complicated, nonreversible and uncontrollable. It is affected by various factors, including the living environment, climate, health, life style, and biological reasons. Age-related facial image processing is being extensively studied, and facial age group classification is one of major research topics in this area.
Examples include age-based facial image retrieval, internet access control, security control and surveillance, biometrics, age-based human-computer interaction (HCI), age prediction for finding missing children, and age estimation based on the result of age groups classification. Age estimation can be done more accurately if it is worked on groups containing a narrower age range. Hence, the age group classification problem is an interesting one that demands further efforts.
Main Ideas
We presented a structured fusion method for facial age group classification as shown in Figure 1. To utilize the structured fusion of shape features and surface features, we introduced the region of certainty (ROC) to not only control the classification accuracy for shape feature based system but also reduce the classification needs on surface feature based system. In the first stage, we design two shape features, which can be used to classify frontal faces with high accuracies. In the second stage, a surface feature is adopted and then selected by a statistical method. The statistical selected surface features combined with a SVM classifier can offer high classification rates. With properly adjusting the ROC by a single non-sensitive parameter, the structured fusion of two stages can provide a performance improvement. In the experiments, we use face images in the public available FGNET and MORPH databases and partition them into three pre-defined age groups. It is observed that the proposed method offers a correct classification rate of 95.1% in FGNET [1] and 93.7% in MORPH [2], which outperforms state-of-the-art methods by a significant margin.
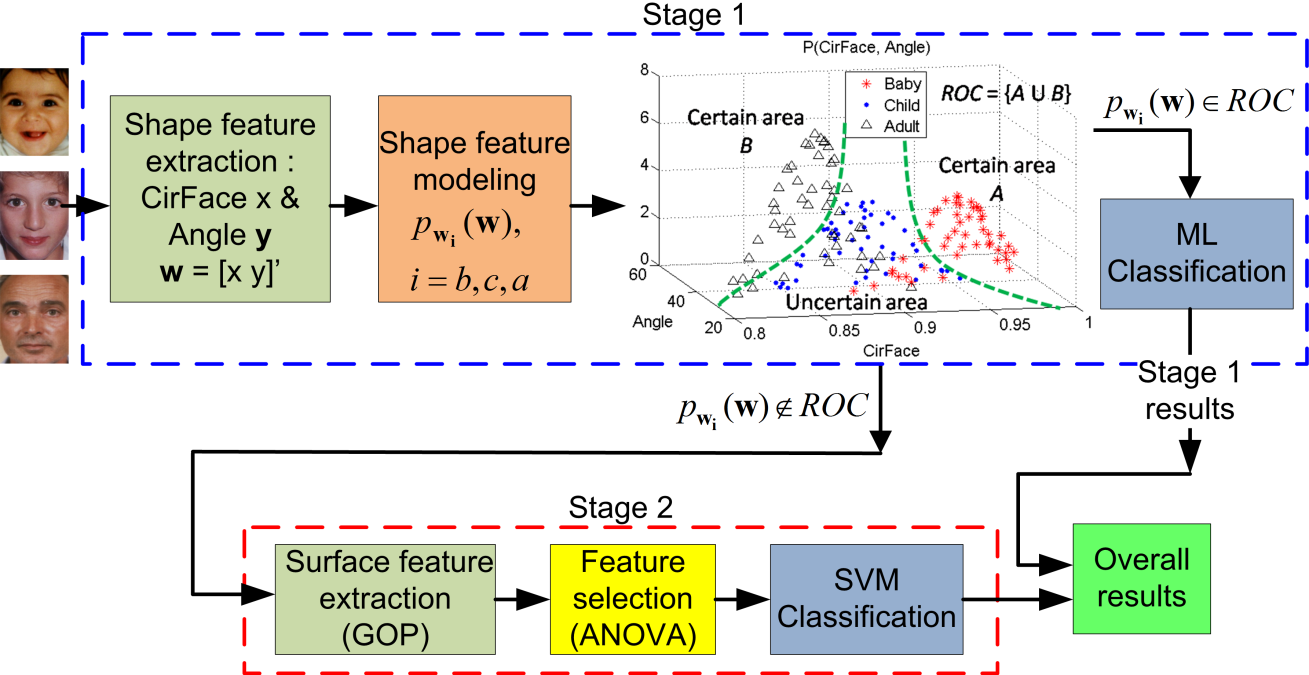
Innovations
Our current work has the following main contributions. First, two new shape-based facial features, “CirFace” and “Angle”, are introduced for age group classification at the first time. Second, a jointly Gaussian distribution is used to model these new shape features and an ML decision rule is chosen based on this model. Third, a feature selection technique is presented in selecting GOP features for complexity reduction and accuracy improvement. Finally, a novel structured fusion system for age group classification system is proposed by designing a region of certainty (ROC) to utilize both shape and surface features, and it outperforms state-of-the-art methods by a significant margin.
Future Challenges
In the future, we will explore other shape/surface features and fuse different shape/surface features together. We will explore the impact of facial landmarks in the proposed shape-based approach. Also, we may consider the effects of the number of pyramid layers for GOP and use/combine other surface-based features. Besides, non-frontal faces will be considered in the future. More than three age groups (i.e., decades of life: 0-9, 10-19, 20-29, 30-39, 40-49, etc.) classification problem will also be an interesting and challenging topic.
References
- [1] The fg-net aging database, http://www.fgnet.rsunit.com/.
- [2] K. Ricanek and T. Tesafaye. Morph: A longitudinal image database of normal adult age-progression. In 7th IEEE International Conference on Automatic Face and Gesture Recognition (FGR’06), pages 341–345, 2006.
- [3] Y. Kwon and N. Lobo. Age classification from facial images. Computer vision and image understanding, 74(1):1–21, 1999.
- [4] X. Geng, Z.-H. Zhou, and K. Smith-Miles. Automatic age estimation based on facial aging patterns. IEEE Transactions on Pattern Analysis and Machine Intelligence, 29(12):2234–2240, 2007.
- [5] A. Lanitis, C. Draganova, and C. Christodoulou. Comparing different classifiers for automatic age estimation. IEEE Transactions on Systems, Man, and Cybernetics, Part B: Cybernetics, 34(1):621–628, 2004.
- [6] Y. Zhang and D.-Y. Yeung. Multi-task warped gaussian process for personalized age estimation. In Computer Vision and Pattern Recognition (CVPR), 2010 IEEE Conference on, pages 2622–2629. IEEE, 2010.
- [7] C. Li, Q. Liu, J. Liu, and H. Lu. Learning ordinal discriminative features for age estimation. In Computer Vision and Pattern Recognition (CVPR), 2012 IEEE Conference on, pages 2570–2577. IEEE, 2012.